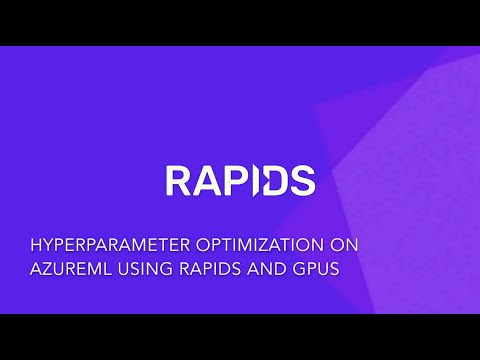
This video tutorial walks through an example of accelerated hyperparameter optimization (HPO) jobs using RAPIDS on Microsoft AzureML. Each job uses RAPIDS dataframe library, cuDF, to load and process 20 million rows of airline arrival and departure data and build a model using RAPIDS machine learning library, cuML. Random Forest is used to predict whether or not a flight will arrive on time. AzureML trains and evaluates models with 100 variations of key parameters in order to find the combination that yields the highest accuracy. In this example, RAPIDS will reduce model training time by 25x, which will allow you to train dozens of models on the GPU, in the same time that it would take to train a model on the CPU.
- Hyperparameter Optimization (HPO) with RAPIDS on Microsoft AzureML and NVIDIA GPUs ( Download)
- How can you automate hyperparameter tuning in Azure Machine Learning ( Download)
- NVIDIA GTC 2020 - Speed Up Data Science Tasks By a Factor of 100+ Using AzureML, DASK and RAPIDS ( Download)
- Learn how to perform auto hyperparameter tuning in Azure ( Download)
- #117: Accelerating ML with RAPIDS by Nvidia AI ( Download)
- Azure Machine Learning (AzureML) Tune Model Hyperparameter ( Download)
- Accelerating MLFlow Hyper-parameter Optimization Pipelines with RAPIDS ( Download)
- 13 Hyperparameter Tuning TensorFlow avec Azure ML ( Download)
- Accelerated Data Science with NVIDIA RAPIDS ( Download)
- Quansight and RAPIDS Push Data Science Performance ( Download)
- ALCF Developer Sessions: High Performance Data Science with RAPIDS ( Download)
- Taking Models to the Next Level with Azure Machine Learning - Henk Boelman - NDC Sydney 2020 ( Download)
- 5. Introduction to cuML ( Download)
- KDD 2020: Hands On Tutorials: Accelerating and Expanding End-to-End Data Science Workflows with ( Download)
- Live- Exploring Nvidia RAPIDS- Open GPU Data Science ( Download)